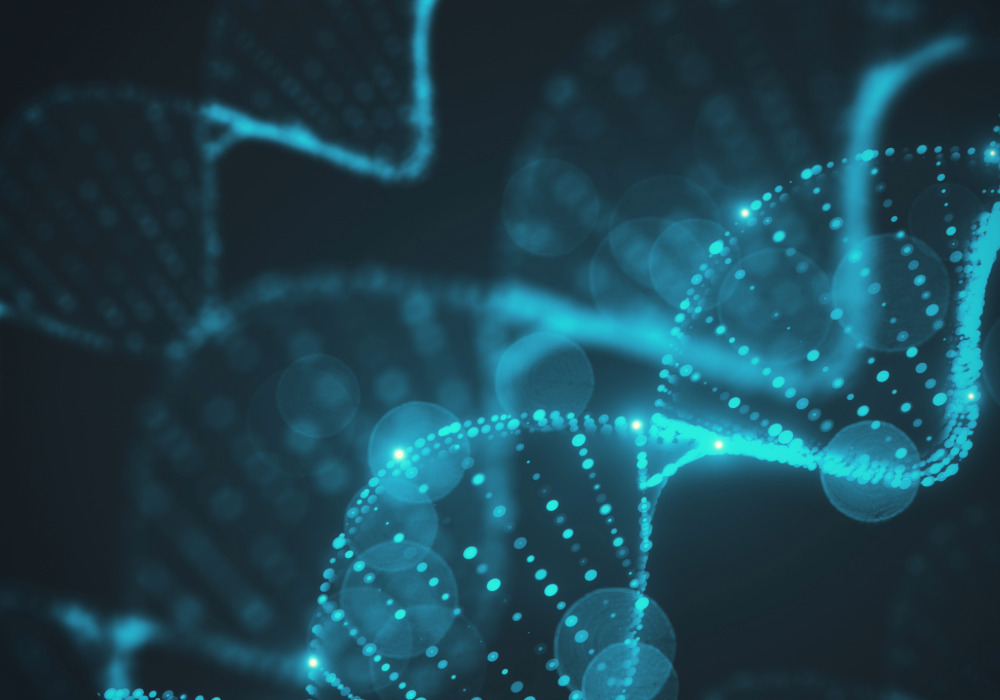
Revista Genética Médica y Genómica
Revista científica online en español que incluye artículos sobre las herramientas y aplicaciones de la Genética y la Genómica en la Medicina.
La revista Genética Médica y Genómica, de ámbito científico nacional e internacional, publica artículos de investigación clínica o básica, revisiones científicas, artículos de opinión, editoriales y comentarios relacionados con la genética y la genómica en el estudio de las enfermedades humanas y sus aplicaciones en la práctica clínica.
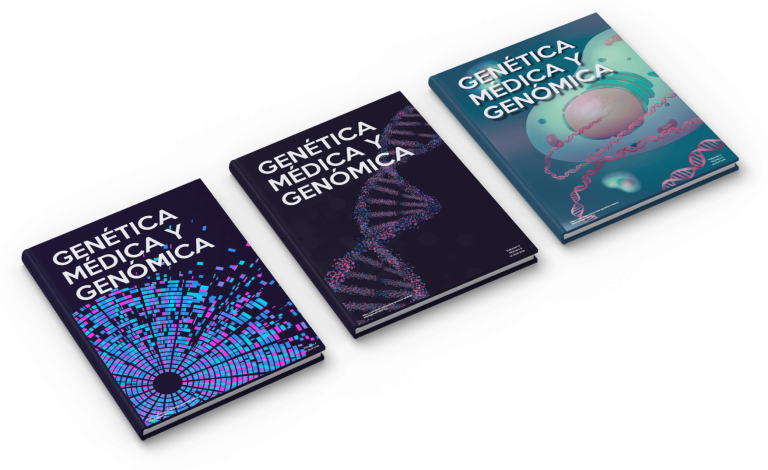
Últimos artículos publicados
La primera revista científica en Genética Médica y Genómica en Español
Genética Médica y Genómica está dirigida a investigadores y profesionales clínicos del área de la Biomedicina, especialmente aquellos enfocados en el área del diagnóstico genético y la medicina genómica de precisión.
ISSN: 2605-0463 Genética Médica y Genómica (Internet)
Publica en Genética Médica y Genómica
La revista científica Genética Médica y Genómica acepta artículos originales para su publicación. Puedes presentar artículos de opinión, comentarios, casos clínicos, artículos de investigación, revisiones científicas, cartas al director o notas metodológicas entre otros manuscritos.
La publicación de trabajos en Genética Médica y Genómica no conlleva ningún coste económico por parte de los autores.
Comité Editorial y Científico
Director
Manuel Pérez Alonso
Universitat de València, España
Editora y redactora
Amparo Tolosa
Diseño y maquetación
Medigene Press S.L, España
Mercedes Aler
Experta en Genética Forense y Criminalística
Esteban Ballestar
Josep Carreras Leukaemia Research Institute
María Blasco
Centro Nacional de Investigaciones Oncológicas (CNIO)
Mª José Calasanz Abinzano
Universidad de Navarra
Ángel Carracedo
Universidad Santiago de Compostela
Manuel Corpas
The Alan Turing Institute, Londres
Cambridge Precision Medicine, Cambridge
University of Westminster, Londres
David de Lorenzo
Centro de Estudios en Genómica y Nutrición – CESGEN Universitat Pompeu Fabra
Pilar Domingo Calap
Instituto de Biología Integrativa de Sistemas Universitat de València
Carmen Escobedo-Lucea
Wyss Institute Harvard University
Fundación de Investigación Hospital General de Valencia (FIHGUV)
Carmen Espinós Armero
Centro de Investigación Príncipe Felipe (CIPF), Valencia
Unidad 763 del CIBER de Enfermedades Raras (CIBERER)-ISCIII
Universitat Politècnica de València
Irene Esteban Marcos
Queen Elisabeth University Hospital, Glasgow
Manel Esteller
Josep Carreras Leukaemia Research Institute, Barcelona
Jaime Font de Mora
Instituto de Investigación Sanitaria IIS-La Fe, Valencia
Enrique Galán Gómez
Universidad de Extremadura Hospital Materno Infantil – Hospital Universitario de Badajoz
Juan de Dios García Díaz
Hospital Universitario Príncipe de Asturias, Madrid
Universidad de Alcalá de Henares, Madrid
José Miguel García Sagredo
Universidad de Alcalá, Madrid
Real Academia Nacional de Medicina de España
Roser González
Universitat de Barcelona
Encarnación Guillén Navarro
Hospital Clínico Universitario Virgen de la Arrixaca IMIB-Arrixaca Universidad de Murcia
CIBER de Enfermedades Raras (CIBERER)-ISCIII
José Luis Ivorra
Premier Research, Madrid
Arturo López Castel
Universitat de València
Adolfo López de Munain Arregui
Hospital Universitario Donostia Instituto Biodonostia
José Antonio López Guerrero
Fundación del Instituto Valenciano de Oncología (IVO)
Carlos López Otín
Universidad de Oviedo
José Antonio Lorente Acosta
Universidad de Granada Centro Pfizer-Universidad de Granada- Junta de Andalucía de Genómica e Investigación Oncológica (GENYO)
Ana Lluch
Universitat de València
Fundación Investigación del Hospital Clínico Universitario de Valencia (INCLIVA)
Gemma Marfany
Universitat de Barcelona
Institut de Biomedicina de la Universitat de Barcelona (IBUB) – Institut de Recerca Sant Joan de Déu (IRSJD)
CIBER de Enfermedades Raras (CIBERER)-ISCIII
Julio César Martín Rodríguez
Iviomics S.L. Instituto Universitario IVI Valencia
Francisco Martínez Castellano
Hospital Universitario y Politécnico la Fe de Valencia
José María Millán
Instituto de Investigación Sanitaria IIS-La Fe
CIBER de Enfermedades Raras (CIBERER)-ISCIII
Mª Dolores Moltó
Universitat de València
Fundación Investigación del Hospital Clínico Universitario de Valencia (INCLIVA)
CIBER de Salud Mental (CIBERSAM)-ISCIII
Lluís Montoliu
Centro Nacional de Biotecnología (CNB-CSIC)
CIBER de Enfermedades Raras (CIBERER)-ISCIII
Lorenzo Montserrat Iglesias
Dilemma Solutions, A Coruña
Federico Vicente Pallardó Calatayud
Universitat de València
Teresa Pampols Ros
Hospital Clinic de Barcelona
Nuria Paricio
Universitat de València
Manuel Pérez Alonso
Universitat de València
Antonio Pérez Aytés
Hospital Universitario y Politécnico la Fe de Valencia
Aurora Pujol
Instituto de Investigación Biomédica de Bellvitge (IDIBELL)
Óscar Puig
Nucleai, New York
Ramiro Quiroga de la Cruz
Hospital Universitario y Politécnico La Fe de Valencia
Feliciano Ramos
Universidad de Zaragoza
Susana Rodríguez-Navarro
Instituto de Biomedicina de Valencia Consejo Superior de Investigaciones Científicas
Jordi Rosell Andreo
Hospital Universitario Son Espases, Palma de Mallorca
Mónica Roselló
Hospital Universitario y Politécnico La Fe de Valencia
Miguel Urioste
Experto en cáncer familiar
Eduardo Vilar Sánchez
MD Anderson Cancer Center, Houston, EE.UU
Contacto
¿Quieres publicar con nosotros? ¿Tienes dudas?
Contacta con nosotros de la manera que prefieras y te responderemos a la mayor brevedad.